27.09.2024
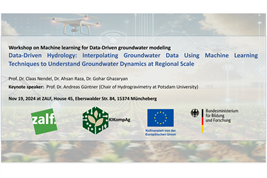
Data-Driven Hydrology: Interpolating Groundwater Data Using Machine Learning Techniques to Understand Groundwater Dynamics at regional scale
Künstliche Intelligenz Kompetenz in der Landwirtschaft (KIKompAg) is a multi-disciplinary project that aims to coordinate and advance the concept for the integration of multisource data, artificial intelligence (AI) and various simulation methods for the cross-scale monitoring of agricultural systems. One of the primary foci is on how machine learning applications can be used to improve our understanding of groundwater dynamics at different scales.
Machine Learning (ML) techniques have widely been used recently in agroecosystem problems. Scientists use these techniques for weed detection, disease control, yield prediction, and climate change impact analysis. However, ML techniques have also great potential to unravel large-scale groundwater supply to crops. In this context, the use of ML involves leveraging advanced algorithms and data analytics to understand and predict the behavior of groundwater systems. By integrating diverse datasets, including satellite observations, in-situ ground observations, and climate data, machine learning helps in creating comprehensive models that provide insights into groundwater dynamics.
Scope
The workshop is designed for researchers in agro-hydrological studies and practitioners who are interested in gaining a
practical introduction to Machine Learning and exploring its
potential applications in groundwater modeling. In the workshop,
theoretical content will be taught and then implemented using practical examples.
The specific aims are to advance the first two protocol-based studies of KIKompAg, which focus on
(1) the downscaling of GRACE-derived GWSA to 1 km2 using the ML approach and identifying the anomaly trends and
(2) the reconstruction of high-resolution groundwater data at 1 km2 spatial resolution using different ML methods and their inter-comparison.
We will examine the use of time series data and conduct several hands-on sessions where participants will have the opportunity to test the developed processing models.
Our learning content:
-
Machine Learning overview with R
-
Preprocessing of data
-
Process monitoring and optimization
-
Performance evaluation of machine learning models
-
Practice-oriented & application-related hands-on
Date: Novmeber 19th, 2024
Place: ZALF, House 45, Eberswalder Str. 84, 15374 Müncheberg
Time duration: One day (9:00 – 16:00)
Format: On-site
Registration:
https://forms.office.com/r/6865c8itR8
Deadline: October 15th, 2024
Contact: ahsan.raza@zalf.de
Workshop Info as PDF